Causal Discovery - What can we learn from heterogeneous noise?
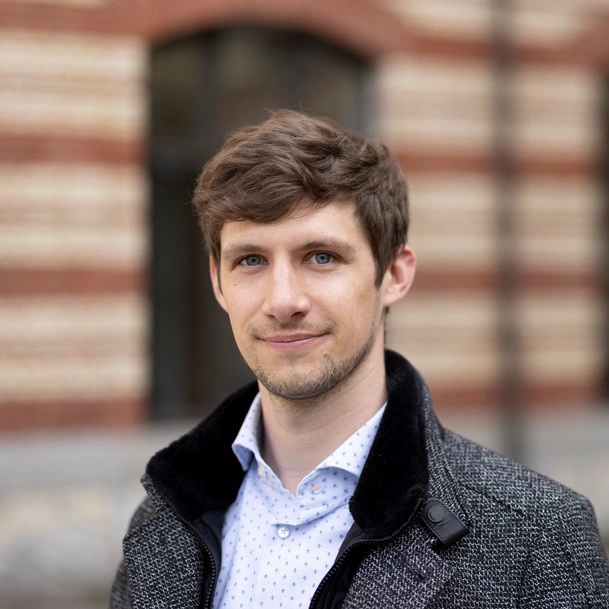
Causal discovery aims to learn causal networks, i.e., directed acyclic graphs (DAGs), from observational data. Although the problem is not feasible in the most general form, as it is not possible to infer causal relations from pure correlations, we can define structural assumptions about the functional relations underlying our observed system to ease this task. One of the earliest works in this direction assumes that all causal relationships are linear and all noise sources can be characterised as non-Gaussian. Under these assumptions we can provably learn causal graphs from observational data—however, they are quite restrictive. In this talk, we relax these assumptions and show that the broader class of location-scale or heteroscedastic noise models (LSNMs) allows for learning causal graphs up to pathological cases. Further, we emphasise that estimation of such functions also plays a key role in causal discovery and discuss several estimators for LSNMs ranging from consistent estimators to Bayesian neural networks.
About Alexander:
Alexander Marx is a professor at TU Dortmund, leading the Causality group at the Research Center for Trustworthy Data Science and Security and the Department of Statistics, and a member of the ELLIS society. His research is at the intersection of causality and machine learning, focusing on causal discovery, causal representation learning, information theory, and Bayesian deep learning. He was a postdoctoral researcher in the Computational Biology Group at ETH Zürich, a postdoc fellow at the ETH AI Center, and part of the Medical Data Science Group. He did his PhD in the Exploratory Data Analysis group affiliated with the CISPA Helmholtz Center for Information Security and the Max Planck Institute for Informatics.