Domain-specific methods for joint embedding self-supervised learning with ultrasound images
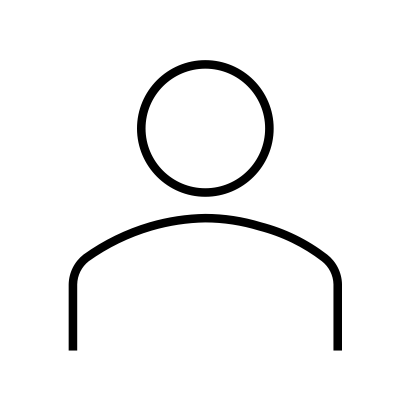
Join the talk at: https://us04web.zoom.us/j/77917991926?pwd=DVVp8yRWGKuXH7cRubIesPYTccprE9.1
Self-supervised learning (SSL) is a strategy for addressing the paucity of labelled data in medical imaging by learning representations from unlabelled images. Contrastive and non-contrastive SSL methods learn representations that are similar for pairs of related images. Such pairs are commonly constructed by randomly distorting the same image twice. We explored different means for defining the similarity relationship between pairs of ultrasound images. First, we investigated the effect of utilizing proximal, distinct images from the same B-mode ultrasound video as pairs for SSL. Additionally, we introduced a sample weighting scheme that increases the weight of closer image pairs and demonstrated how it can be integrated into SSL objectives. This method surpassed previous ultrasound-specific contrastive learning methods’ average test accuracy on COVID-19 classification with the POCUS dataset by ≥ 1.3%. Second, we designed an ultrasound-specific data augmentation pipeline designed to encourage pretrained models to learn ultrasound-specific invariance relationships. Composed of novel and pre-existing SSL transformations, the usage of the novel pipeline led to a test AUC of 0.903 for the task of pleural effusion detection, which was 0.0303 greater than a standard set of transformations. The results indicated that domain-specific transformations can improve ultrasound self-supervised models for some tasks, but that other transformations that may produce semantically inconsistent pairs may be required in addition to achieve top performance on other tasks.
About Blake:
Blake is a PhD Candidate at the Cheriton School of Computer Science, University of Waterloo.