Causally Aware Machine Learning
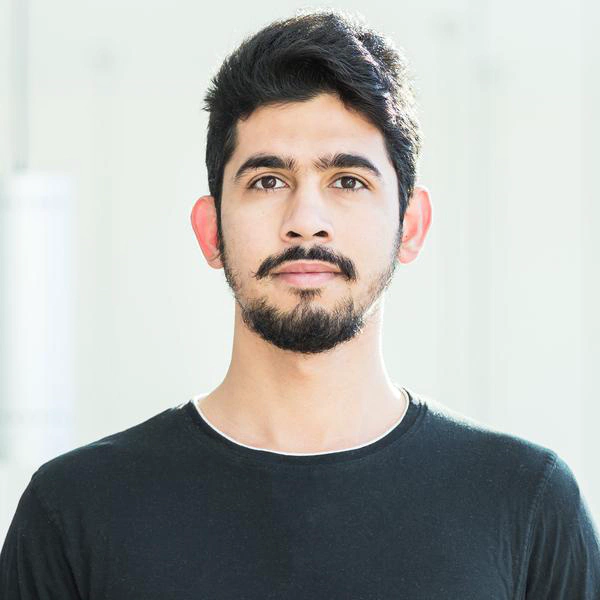
Over the years, machine learning (ML) has significantly advanced in efficiently uncovering intricate patterns within extensive datasets. While ML algorithms adeptly detect patterns and correlations within data, their primary focus is on capturing statistical relationships between variables. It is widely recognized, however, that a strong correlation does not necessarily imply causation, which limits the generalization capability of ML approaches. To address these limitations, causal learning emerges as a promising avenue, emphasizing not only the identification of underlying generation mechanisms but also the identification of the correct set of variables corresponding to true “causes” of our target of interest. Only with the precise specification of causal variables can we hope to learn generalizable mechanisms. In the first segment of the presentation, I will provide a brief introduction to causal structure learning and its potential benefits for (supervised) ML algorithms. I will introduce the paradigms associated with causal structure learning and examine some significant challenges encountered in the field in recent years. In the latter part, I will delve into strategies for systematically addressing these challenges to develop practical causal structure learning solutions better suited for real-world applications. We will see approaches capable of offering deeper causal insights compared to conventional causal structure learning algorithms, learning underlying causal structures from heterogeneous data, and ensuring our causal discovery approaches are federated and privacy-preserving. Concluding the presentation, we will look at several ongoing challenges and potential avenues for exciting future research directions.
Visit his website for more: https://sites.google.com/view/mian/
About Osman: Osman is a final-year PhD student at the University of Saarland and the CISPA Helmholtz Center for Information Security in the Exploratory Data Analysis group of Prof. Dr. Jilles Vreeken. His research focusses on causal discovery and inference, and how it can be used to make machine learning robust, including work on privacy-preserving federated causal discovery algorithms under mild assumptions such that these methods can actually be applied to real-world scenarios.